Table of Contents
The global machine learning market, valued at USD 36.73 billion in 2022, is projected to grow at a 34.8% CAGR from 2023 to 2030. This surge highlights the increasing adoption of machine learning across sectors like healthcare, finance, retail, and logistics, driving enhanced efficiency and innovation.
Machine Learning (ML) can be defined as the scientific study of algorithms that a computer uses to complete predefined tasks without human intervention. ML relies on patterns, movements, and inferences from the objects in its provided tasks. While we already see ML use in our day-to-day activities like face detection, speech recognition, trading algorithms, and online streaming recommendations, this technology can be used in modern-day warehouses to elevate automation and increase productivity.
ML helps improve the efficiency of a warehouse but also aids in organizing operations, allowing warehouse staff to focus on product quality and customer experience. Let’s examine how machine learning can revolutionize the modern-day warehouse experience.
Practical Applications of Machine Learning in Core Areas
Labour Management Systems
In warehouse management, ML can replace traditional planning and optimization tools that rely on process modeling or engineering. For instance, consider labour management systems based on engineered labor standards (ELS). An ELS-based system is explicitly programmed to calculate expected work completion times for a given task using predefined numbers and variables, requiring substantial upfront investment and measurements. Machine learning, on the other hand, simplifies this task by analyzing streams of operational data without the need for upfront engineering and numbers. Data can arrive through various sources, such as mobile devices and automation systems, even Warehouse Control Systems (WCS).
Product Receiving
Receiving new products in a warehouse can also be streamlined with ML. ML can analyze the current location of items in a warehouse and, when new shipments arrive, match them to the correct location, thereby reducing time consumption, and mistakes, and increasing operational efficiency. Additionally, ML can direct manual workers to the shortest route to reach a specific point in the warehouse, saving time.
Order Picking
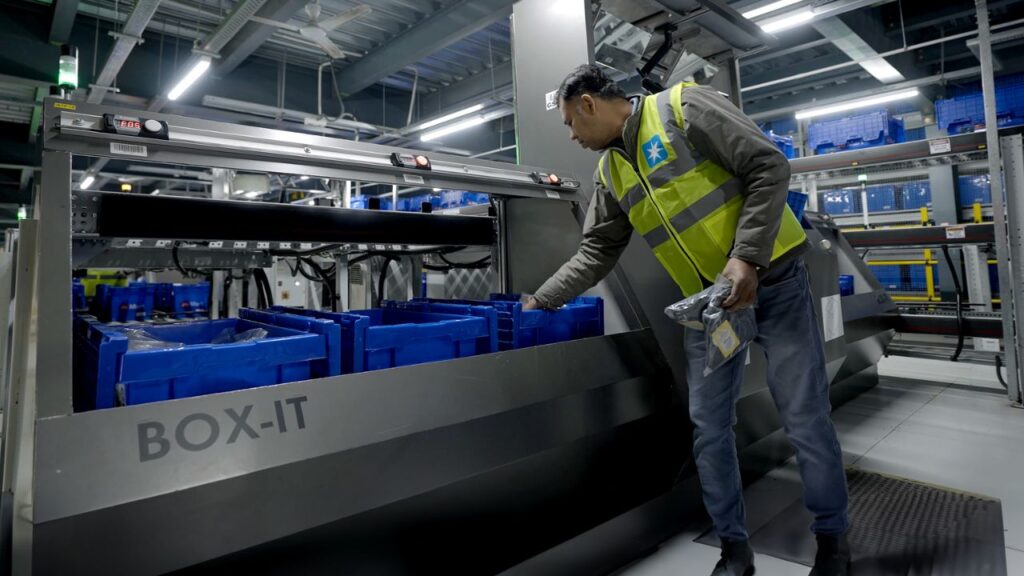
Picking is one of the highest visibility tasks in a warehouse. According to a report by Adaptalift, order picking accounts for as much as 55% of operating costs within a warehouse, directly impacting customer satisfaction levels. ML can optimize picking by reducing the number of steps in the process, thus lowering error margins and damages. While handling multiple orders, ML can analyze the orders in the system and arrange the most direct path, simultaneously separating orders efficiently.
Inventory Management
Inventory management is another critical aspect of modern warehouses that requires meticulous attention to achieve supply chain efficiencies. Machine learning can enhance inventory optimization, especially for companies with multiple warehouses. ML can consider multiple independent variables that might cause errors or delays in managing inventory and provide suitable suggestions and solutions to manage stock efficiently.
Internet of Things (IoT) Integration
The use of IoT inside warehouses can further enhance efficiency. Implementation of sensors, RFID tags, device-to-device communication, and other forms of connectivity can help manage daily tasks seamlessly. ML can process data collected by IoT sensors, extending the life of machineries like conveyor belts, shuttles, and navigation-driven mobile robots, and even detect wear and tear on AGVs and other smaller delivery bots.
The Future of Warehousing
In today’s warehouses, robots often work alongside humans. However, ML can enhance the precision of robots, potentially eliminating the need for human intervention. A future warehouse powered by ML will be a fully automated, error-free space, completing all tasks and projects on time with 100% accuracy. This vision of the future promises to revolutionize warehouse operations, setting new standards in productivity and efficiency.
By integrating ML into warehouse operations, businesses can not only elevate automation but also optimize various processes, leading to significant improvements in productivity, accuracy, and overall operational performance. The future of warehousing, driven by machine learning, is indeed promising and bound to redefine industry standards.
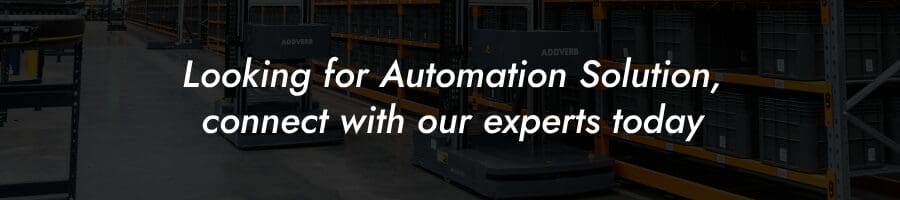
FAQ
What role does machine learning play in modern warehouses?
Machine learning optimizes operations by analyzing data to improve inventory management, demand forecasting, and resource allocation.
How can machine learning improve inventory accuracy?
It analyzes historical data and real-time inputs to predict stock levels, reducing errors and ensuring better stock control.
Can machine learning enhance warehouse efficiency?
Yes, by automating repetitive tasks, optimizing routes for autonomous vehicles, and improving picking accuracy.
How does machine learning help in demand forecasting?
It processes vast amounts of data to identify patterns and trends, enabling more accurate predictions of future demand.
What are the benefits of using machine learning for predictive maintenance?
It predicts equipment failures before they occur, reducing downtime and maintenance costs.